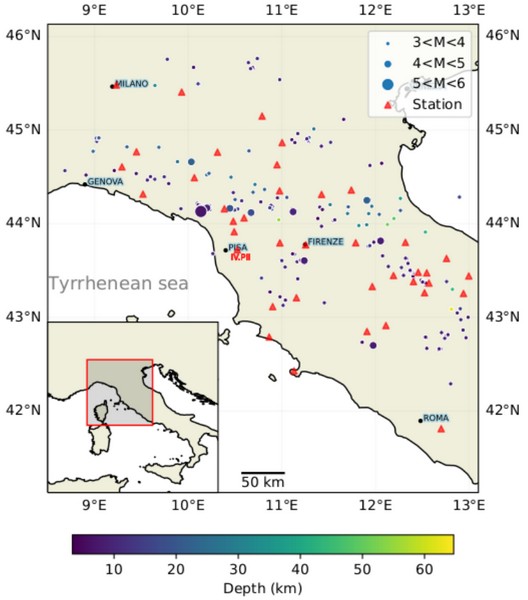
In a recent study we showed that convolutional neural networks (CNNs) applied to network seismic traces can be used for rapid prediction of earthquake peak ground motion intensity measures (IMs) at distant stations using only recordings from stations near the epicenter. The predictions are made without any previous knowledge concerning the earthquake location and magnitude. This approach differs significantly from the standard procedure adopted by earthquake early warning systems (EEWSs) that rely on location and magnitude information. In the previous study, we used 10 s, raw, multistation (39 stations) waveforms for the 2016 earthquake sequence in central Italy for 915 M ≥ 3.0 events (CI dataset). The CI dataset has a large number of spatially concentrated earthquakes and a dense network of stations. In this work, we applied the same CNN model to an area of central western Italy. In our initial application of the technique, we used a dataset consisting of 266 M ≥ 3.0 earthquakes recorded by 39 stations. We found that the CNN model trained using this smaller-sized dataset performed worse compared to the results presented in the previously published study. To counter the lack of data, we explored the adoption of ‘transfer learning’ (TL) methodologies using two approaches: first, by using a pre-trained model built on the CI dataset and, next, by using a pre-trained model built on a different (seismological) problem that has a larger dataset available for training. We show that the use of TL improves the results in terms of outliers, bias, and variability of the residuals between predicted and true IMs values. We also demonstrate that adding knowledge of station relative positions as an additional layer in the neural network improves the results. The improvements achieved through the experiments were demonstrated by the reduction of the number of outliers by 5 per cent, the residuals R median by 39 per cent and their standard deviation by 11 per cent.