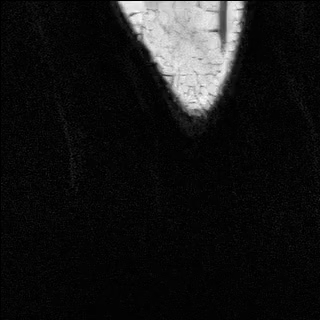
Background and objectives: A radiologist’s work in detecting various injuries or pathologies from radiological scans can be tiresome, time consuming and prone to errors. The field of computer-aided diagnosis aims to reduce these factors by introducing a level of automation in the process. In this paper, we deal with the problem of detecting the presence of anterior cruciate ligament (ACL) injury in a human knee. We examine the possibility of aiding the diagnosis process by building a decision- support model for detecting the presence of milder ACL injuries (not requiring operative treatment) and complete ACL ruptures (requiring operative treatment) from sagittal plane magnetic resonance (MR) volumes of human knees. Methods: Histogram of oriented gradient (HOG) descriptors and gist descriptors are extracted from manually selected rectangular regions of interest enveloping the wider cruciate ligament area. Performance of two machine-learning models is explored, coupled with both feature extraction methods: support vector machine (SVM) and random forests model. Model generalisation properties were determined by performing multiple iterations of stratified 10-fold cross validation whilst observing the area under the curve (AUC) score. Results: Sagittal plane knee joint MR data was retrospectively gathered at the Clinical Hospital Centre Rijeka, Croatia, from 2007 until 2014. Type of ACL injury was established in a double-blind fashion by comparing the retrospectively set diagnosis against the prospective opinion of another radiologist. After clean up, the resulting dataset consisted of 917 usable labelled exam sequences of left or right knees. Experimental results suggest that a linear-kernel SVM learned from HOG descriptors has the best generalisation properties among the experimental models compared, having an area under the curve of 0.894 for the injury-detection problem and 0.943 for the complete-rupture-detection problem. Conclusions: Although the problem of performing semi-automated ACL-injury diagnosis by observing knee-joint MR volumes alone is a difficult one, experimental results suggest potential clinical application of computer- aided decision making, both for detecting milder injuries and detecting complete ruptures.
The dataset used in this research is publicly available: http://www.riteh.uniri.hr/~istajduh/projects/kneeMRI/