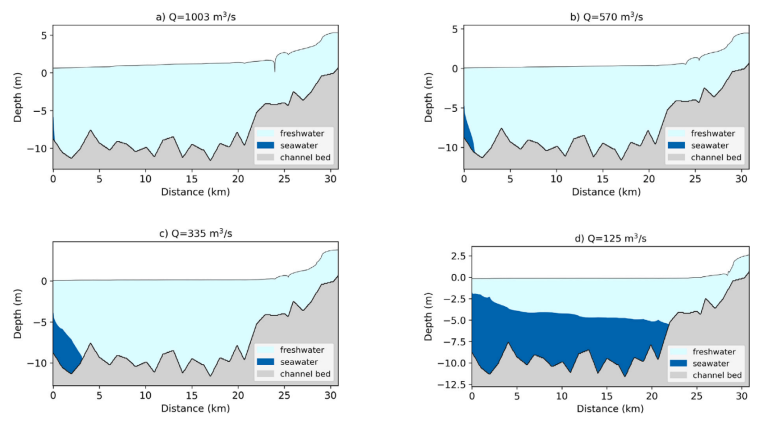
The challenges of managing water resources in tidal rivers, exacerbated by climate change and anthropogenic impacts, require innovative approaches for accurate estimation of hydrological parameters. In tidal rivers and estuaries, water levels depend primarily on river discharge and tidal dynamics. Microtidal estuaries are particularly complex due to the strong stratification and two-layer structure, which also affect the water level. This study investigates the potential of machine learning (ML) models for estimating discharge in the Neretva River, Croatia, using only water level data from multiple stations. Comparative analyzes were performed between simple supervised models – Decision Tree (DT), Random Forest (RF), Support Vector Regression (SVR), Light Gradient Boosting Machine (LGBM), Extreme Gradient Boosting (XGB) – and time series models – Long Short-Term Memory (LSTM) and LSTM-Attention. Both simulated and measured data sets were used for this purpose. The results show that time series models perform satisfactorily in the assessment of discharge and overcome the challenges faced by simple supervised models, especially under high flow scenarios. Overall, LSTM-Attention proves to be the best model when analyzing all error metrics with superior performance over the entire range of discharge values. It surpasses the overall LSTM model performance, with a percentage increase of above 9% in RMSE and MAE, above 0.2% in NSE, and above 0.1% in R for both simulated and measured datasets.