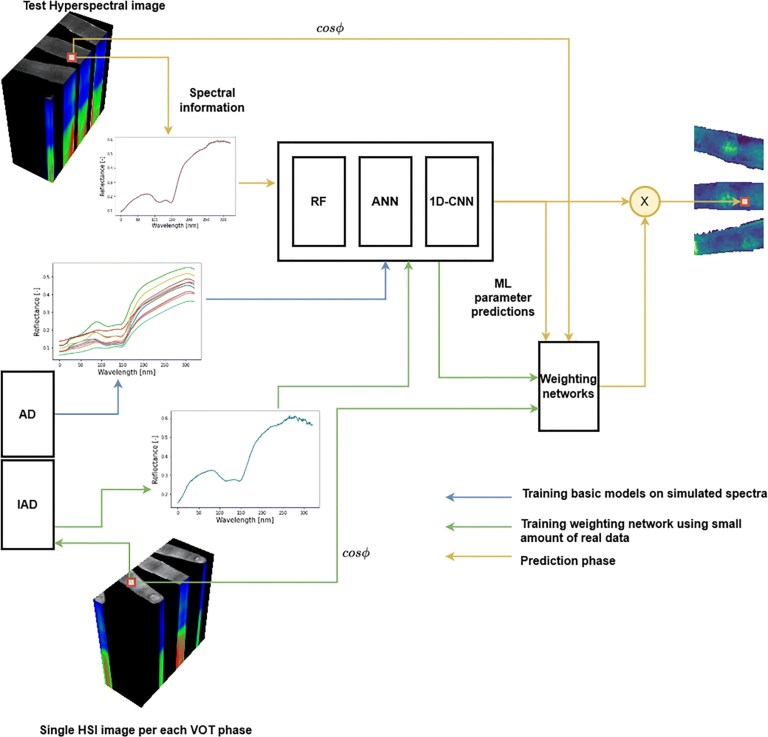
Noninvasive assessment of skin structure using hyperspectral images has been intensively studied in recent years. Due to the high computational cost of the classical methods, such as the inverse Monte Carlo (IMC), much research has been done with the aim of using machine learning (ML) methods to reduce the time required for estimating parameters. This study aims to evaluate the accuracy and the estimation speed of the ML methods for this purpose and compare them to the traditionally used inverse adding-doubling (IAD) algorithm. We trained three models – an artificial neural network (ANN), a 1D convolutional neural network (CNN), and a random forests (RF) model – to predict seven skin parameters. The models were trained on simulated data computed using the adding-doubling algorithm. To improve predictive performance, we introduced a stacked dynamic weighting (SDW) model combining the predictions of all three individually trained models. SDW model was trained by using only a handful of real-world spectra on top of the ANN, CNN and RF models that were trained using simulated data. Models were evaluated based on the estimated parameters’ mean absolute error (MAE), considering the surface inclination angle and comparing skin spectra with spectra fitted by the IAD algorithm. On simulated data, the lowest MAE was achieved by the RF model (0.0030), while the SDW model achieved the lowest MAE on in vivo measured spectra (0.0113). The shortest time to estimate parameters for a single spectrum was 93.05 μs. Results suggest that ML algorithms can produce accurate estimates of human skin optical parameters in near real-time.