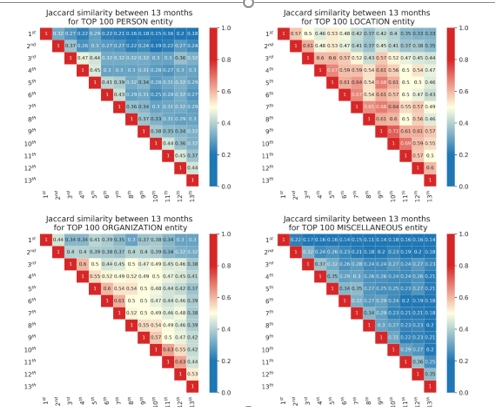
Background: Online media plays an important role in public health emergencies and serves as a communication platform. Infoveillance of online media during the COVID-19 pandemic is an important step toward a better understanding of crisis communication.
Objective: The goal of this study is to perform a longitudinal analysis of the COVID-19 related content based on natural language processing methods.
Methods: We collected a dataset of news articles published by Croatian online media during the first 13 months of the pandemic. Firstly, we test the correlations between the number of articles and the number of new daily COVID-19 cases. Secondly, we analyze the content by extracting the most frequent terms and apply the Jaccard similarity. Next, we compare the occurrence of the pandemic-related terms during the two waves of the pandemic. Finally, we apply named entity recognition to extract the most frequent entities and track the dynamics of changes during the observed period.
Results: The results show there is no significant correlation between the number of articles and the number of new daily COVID-19 cases. Furthermore, there are high overlaps in the terminology used in all articles published during the pandemic with a slight shift in the pandemic-related terms between the first and the second wave. Finally, the findings indicate that the most influential entities have lower overlaps for the identified persons and higher overlaps for locations and institutions.
Conclusions: Our study shows that online media has a prompt response to the pandemic with a large number of COVID-19 related articles. There is a high overlap in the frequently used terms across the first 13 months, which may indicate the narrow focus of reporting in certain periods. However, the pandemic-related terminology is well covered.