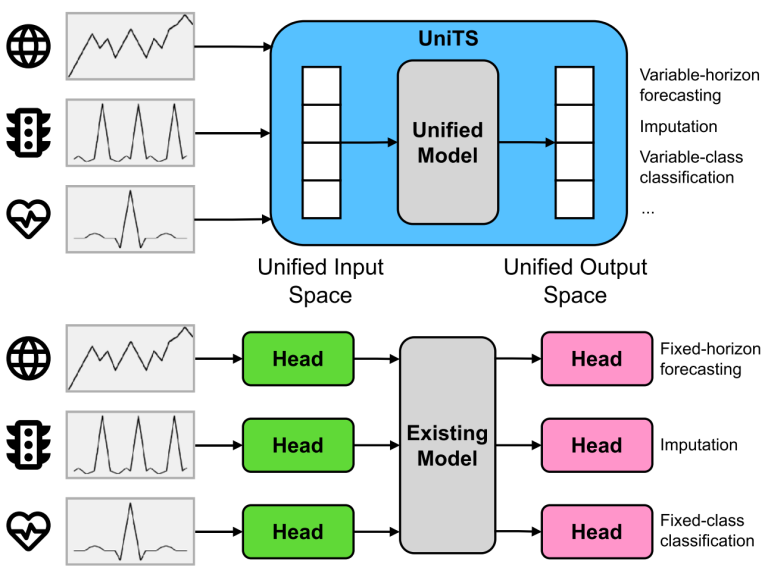
Monitoring and predicting personal watercraft trajectories is a novel and largely unexplored research area where any development is valuable for various rental services. Unlike existing work focused on specific maritime routes, this study introduces a location-agnostic deep-learning approach capable of generalizing across diverse environments. This is achieved by using an innovative preprocessing approach including offset and scaling. A novel Long Short-Term Memory (LSTM) bidirectional and convolutional model developed by the authors for binary peptide classification was adapted to accommodate the forecasting of continuous values. By leveraging Recurrent Neural Network (RNN) architectures with LSTM and Gated Recurrent Unit (GRU) layers, and cutting-edge attention-based and foundation models, we benchmark trajectory forecasting performance using real-world data from 1282 rental sites worldwide. Most notably, we extend the applicability of the listed models and the original LSTM bidirectional and convolutional models to maritime trajectory estimation, eliminating the need for training separate models for different locations while achieving superior predictive accuracy. Our results demonstrate that foundation models and attention mechanisms significantly outperform traditional methods, offering a robust and scalable solution for watercraft trajectory forecasting. These findings pave the way for intelligent monitoring systems that enhance maritime safety and operational efficiency.