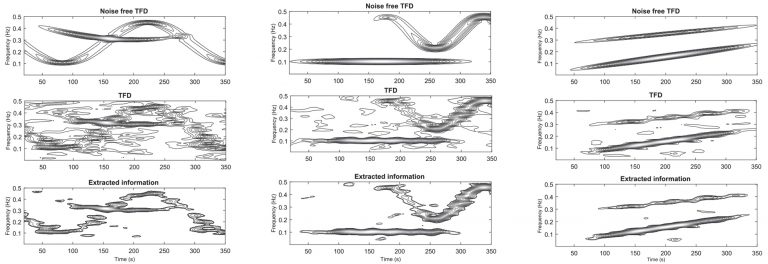
This paper proposes an automatic method for extraction of useful information content from time-frequency distributions of nonstationary signals heavily corrupted by additive noise. The proposed method, which does not require prior knowledge of the signal, initially performs a one-dimensional clustering of the time-frequency distribution aimed at segmenting it into a fixed number of classes. This procedure points out basic structural differences of noise and signal components (i.e., useful information) in the time-frequency plane. In fact, noise presents a flat spectrum with large time-frequency supports, while signal components are narrow energy ridges. The time-frequency supports are estimated for each of the obtained classes and used as input for an algorithm aimed at discriminating “noise” affine classes, containing mainly non-zero coefficients located outside the components time-frequency supports, from “useful information” affine classes, containing mainly coefficients inside time-frequency supports of the signal components. Simulations show the superiority of the proposed algorithm, mainly in terms of error rate reduction of classified time-frequency coefficients, compared to hard amplitude thresholding methods, as well as to a recently proposed method for information content extraction based on adaptive thresholding, which is also outperformed in terms of computational complexity and execution time (by up to 43 and 28 times, respectively).
The research was conducted at the Faculty of Engineering, University of Rijeka (www.riteh.uniri.hr).