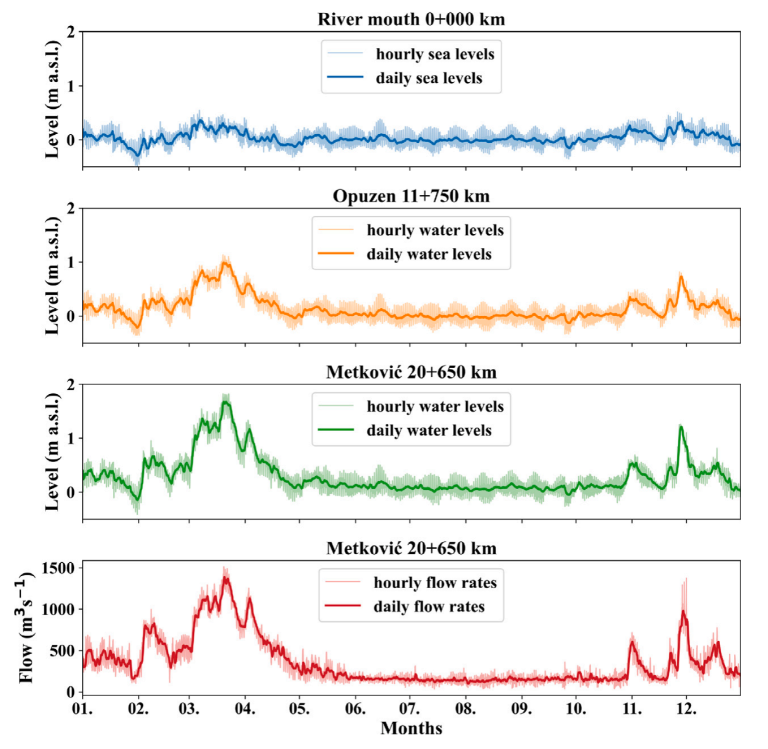
Understanding the dynamics of tidal rivers and estuaries is critical for reliable water management. Recently, the use of Machine Learning (ML) has increased in favor of hydrologic and hydraulic models. The advantages of ML over physically based models are most evident in modeling complex and nonlinear hydrologic processes and inverse problems. This study provides a critical review of ML approaches for forecasting, reconstruction, and establishment of stage-discharge relationships in tidal rivers and estuaries characterized by nonlinear interaction between the river and coastal processes. Gaps in this research area and the limited number of stage-discharge studies are identified and explained. The advantages and limitations of each approach are discussed from a critical perspective, and suggestions are made for future research directions. Advanced Recurrent Neural Networks (RNNs) and hybrid modeling systems combining physically based models with ML appear to be the most promising approaches for modeling complex physical processes in these environments.