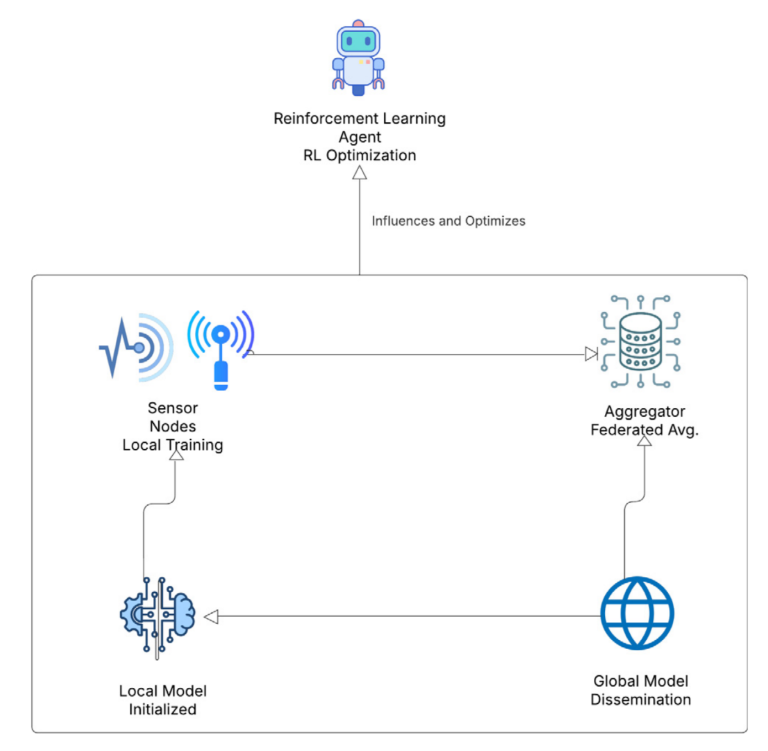
Improved frameworks for delivering both intelligence and effectiveness under strict constraints on resources are required due to the Internet of Things’ (IoT) devices’ rapid expansion and the resulting increase in sensor-generated data. In response, this research considers a joint learning-offloading optimization approach and presents an improved framework for energy-efficient distributed intelligence in sensor networks. Our method dynamically allocates computational tasks across resource-constrained sensors and more powerful edge servers through incorporating Federated Learning (FL) with adaptive offloading techniques. This allows collaborative model training across IoT devices. We suggest a multi-objective optimization problem that simultaneously maximizes learning accuracy and convergence time and minimizes energy usage with the objective to solve the dual issues of energy consumption and model performance. To create energy-efficient distributed intelligence in IoT sensor networks, our suggested framework combines FL, Digital Twin (DT), and sophisticated Reinforcement Learning (RL)-based decision-making engine. In order to predict short-term system dynamics, the DT uses linear regression and moving averages for predictive analytics based on real-time data from sensor nodes, such as battery levels, CPU loads, and network latencies. A Dueling Double Deep Q-Network (D3QN) agent with Prioritized Experience Replay (PER) and multi-step returns is directed by these predictions and dynamically chooses between offloading and local processing depending on the operating environment. According to experimental data, our method effectively keeps final battery levels over 85% while allowing the offloading to reduce local CPU drain. We compare the proposed framework with two baseline methods. The evaluation results show that the pure local strategy obtains a slightly increased average battery level, about 91%, but never offloads tasks, the naïve offload method maintains a lower average battery level, about 70%, than our RL agent’s converged policy, about 85%.