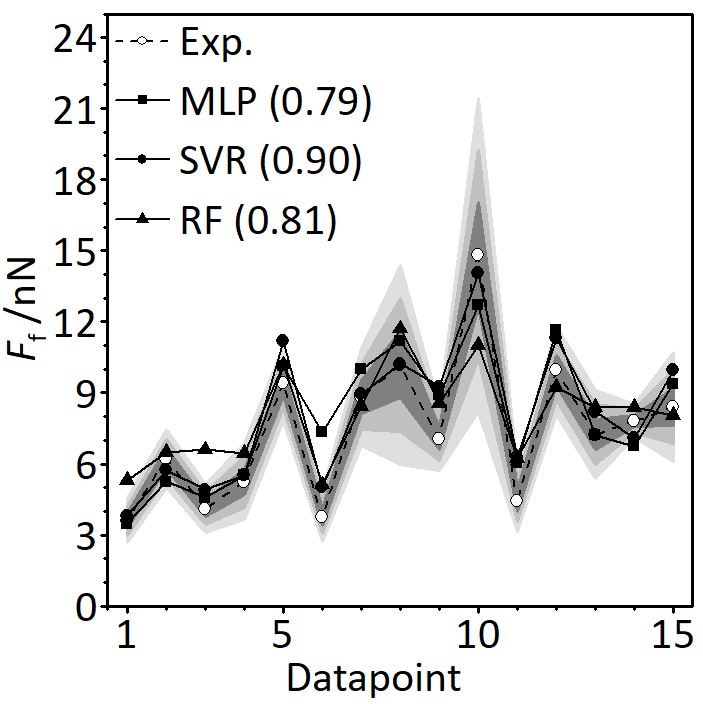
Modelling of nanoscale friction presents a long-lasting challenge. In fact, while there are several generalised models that provide good results for macro- and micro-scale friction, due to the complex concurrent physicochemical interactions in nanoscale contacts, when modelling nanoscale friction there is a clear lack of reliable predicting tools. The modelling methodology proposed in this work is based on the recently performed multidimensional experimental measurements of thin-films’ nanoscale friction, where the concurrent effects of several process parameters are considered. Due to the stochastic nature of the considered phenomena, conventional regression methods yield poor predictive performances. A machine learning (ML) numerical paradigm is hence proposed. Via a comparative study it is hence shown that, while the best typical regression models result in coefficients of determination (R2) of the order of 0.3, the predictive performances of the used ML models, depending on the considered sample, yield R2 in the range from 0.54 to 0.9. The developed models provide also new insights into the functional dependence of the variable process parameters, but also sound basis for future extensions of existing friction models to the nanometric range.