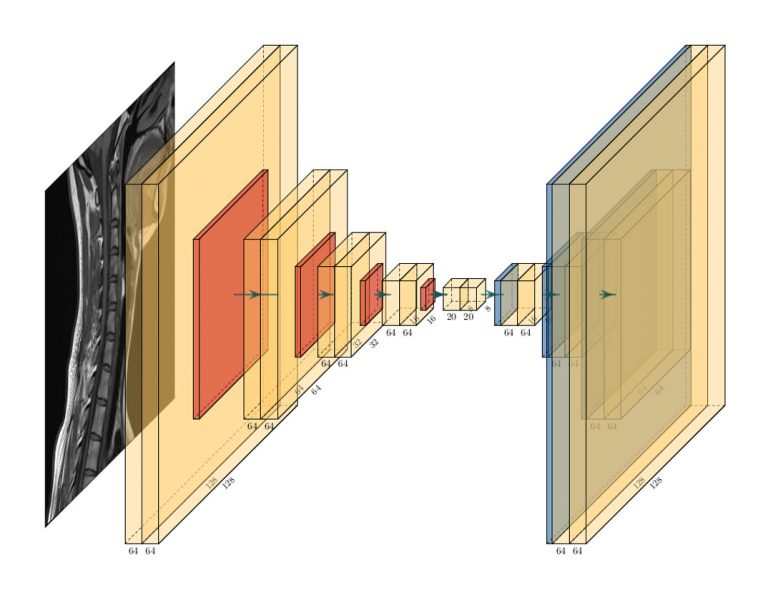
Medical radiology is often used in clinical analysis to establish a medical diagnosis in a non-invasive manner. By considering the morphological properties of the observed area, clinicians can determine the presence of an injury or a disease without the need for invasive surgery. The purpose of computer-aided diagnosis (CAD) is to help physicians with interpreting the recorded data. Recently, the use of machine learning techniques has begun to grow rapidly in this area, increasing the accuracy of manually-crafted models, or even entirely replacing them. A recent apparent increase in the use of these techniques in medical image analysis has been facilitated due to a wider availability of data, increased computer processing power, and a more significant progress achieved through the use of deep-learning techniques in machine vision applications. This development, in turn, has created the potential for introducing significant changes to biology and medicine, both in the laboratory and in the clinic. One of the essential advantages of machine-learning techniques, in comparison to the filtering techniques, in medicine, lies in their ability to unearth new procedures for diagnosing diseases, such that have been inconceivable so far. Additionally, due to the complexity of modelling specific conditions, closely related to patient’s characteristics, machine-learning techniques are increasingly becoming an essential factor in the implementation of personalized medicine in the clinic. The proposed research will advance existing knowledge concerning modelling for medical image analysis, by introducing a common transfer-learning platform for building more accurate predictive models, intended to automate disease and injury detection procedures in medical CAD systems, ultimately resulting in improved health care.
The project is conducted at RITEH.