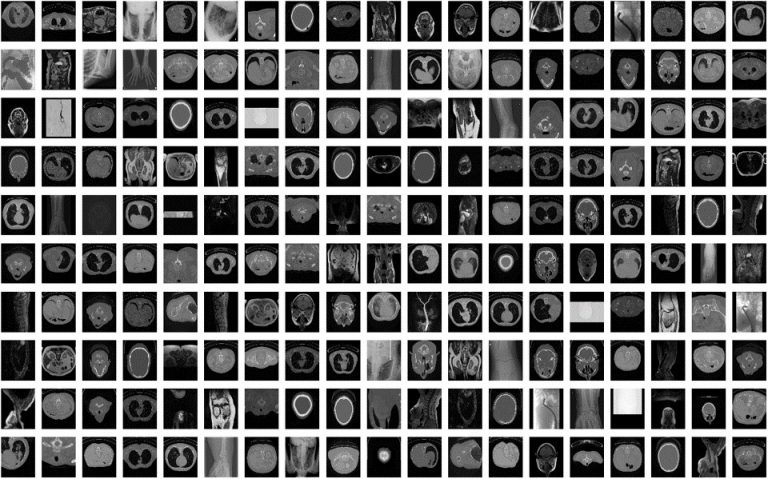
Clinical decision-support systems are often built by manually gathering, formalising and implementing specialist knowledge. Therefore, they are limited by existing human knowledge concerning modelling clinical conditions, diagnosis and therapy, and can be inaccurate because of variations and complexity inherent to medical data. In order to circumvent these limitations, following an obvious growth of publicly available collections of medical-radiology diagnostic images, machine learning has become an irreplaceable tool for solving various problems concerning radiology image analysis.
The goal of this project is to utilise the strength of contemporary findings in the machine-learning and computer-vision field for building quality models for: 1) automating the process for radius bone fracture detection and localisation from arm radiogram, and; 2) automating the process for early diagnosis of arthritis from multimodal (hyperspectral, thermographic, 3D) scans of patient’s hand. This will be achieved through understanding the process of clinical interpretation in medical diagnostics, developing matching physical models of mentioned phenomena for feature extraction and generating synthetic data, whilst using large collections of labelled data for learning models for tissue localisation/segmentation and detection/classification of said phenomena.
The project is conducted at RITEH.