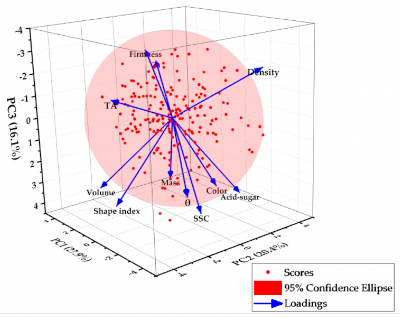
As a result of declining natural resources and growing populations, perishable food chains face the challenge of increasing food handling efficiency and minimizing post-harvest damage. The project proposes an SmaCC system based on the application of in-depth data flow analysis procedures for the analysis of sensory stream data. The data is generated by the latest sensor technology in the task of monitoring the logistical conditions in the cold food chain with the aim of extending the shelf life of food. The large amount of data produced in the cold chain in the form of fast streams is influenced by the phenomenon of change. Due to the consequent lack of stationarity in the distribution of flow data, there is a need for efficient and scalable algorithms for online analysis that are able to adapt to such changes (concept drift). The task of predicting is to detect these rare events. As these events are rarely observed, the prediction task has insufficiently balanced data. To classify the data flow, the SmaCC system will use a cost sensitive learning method that is more time-efficient for data flows than sampling techniques. The SmaCC system will be based on the latest in-depth data flow analysis procedures, for example incremental support vector machine, evolving pulse neural networks, genetic algorithms, group learning.
The project is conducted at the Department of Informatics, University of Rijeka (www.inf.uniri.hr).